Other useful Python packages
Contents
Other useful Python packages#
Announcements#
CAPES and survey available!
Goals of this lecture#
Review of course: what we’ve learned this quarter.
Overview of other useful Python packages:
seaborn
: easily and quickly make data visualizations.scipy
: tools for statistical analyses.nltk
: tools for Natural Language Processing, like sentiment analysis.
What we’ve learned#
Reflect on the very first day of class: most of you had never programmed before!
Now you know:
How to use Jupyter notebooks.
How to write
if
statements andfor
loops.How to create custom functions.
How to read in files of various types.
How to work with tabular data using
pandas
.
That’s a lot for ten weeks!
Data visualization with seaborn
#
What is data visualization?#
Data visualization refers to the process (and result) of representing data graphically.
CSS 2 will dedicate much more time to this.
Today: introduction to
seaborn
.
import matplotlib.pyplot as plt # conventionalized abbreviation
import pandas as pd
import seaborn as sns
%matplotlib inline
%config InlineBackend.figure_format = 'retina'
/opt/anaconda3/lib/python3.9/site-packages/scipy/__init__.py:146: UserWarning: A NumPy version >=1.16.5 and <1.23.0 is required for this version of SciPy (detected version 1.26.2
warnings.warn(f"A NumPy version >={np_minversion} and <{np_maxversion}"
Example dataset#
## Load taxis dataset
df_taxis = sns.load_dataset("taxis")
len(df_taxis)
6433
df_taxis.head(2)
pickup | dropoff | passengers | distance | fare | tip | tolls | total | color | payment | pickup_zone | dropoff_zone | pickup_borough | dropoff_borough | |
---|---|---|---|---|---|---|---|---|---|---|---|---|---|---|
0 | 2019-03-23 20:21:09 | 2019-03-23 20:27:24 | 1 | 1.60 | 7.0 | 2.15 | 0.0 | 12.95 | yellow | credit card | Lenox Hill West | UN/Turtle Bay South | Manhattan | Manhattan |
1 | 2019-03-04 16:11:55 | 2019-03-04 16:19:00 | 1 | 0.79 | 5.0 | 0.00 | 0.0 | 9.30 | yellow | cash | Upper West Side South | Upper West Side South | Manhattan | Manhattan |
Histograms#
Histograms are critical for visualizing how your data distribute.
Used for continuous variables.
sns.histplot(data = df_taxis, x = "distance", alpha = .5, bins = 20)
<AxesSubplot:xlabel='distance', ylabel='Count'>
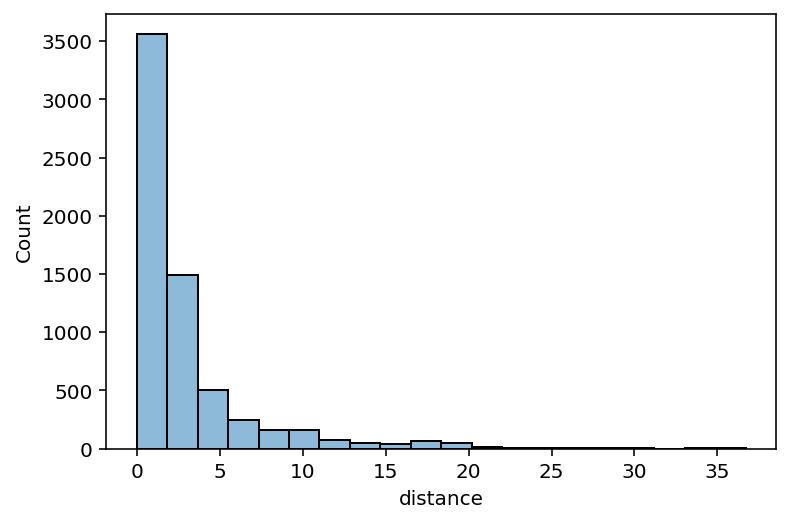
Scatterplots#
Scatterplots are useful for visualizing how two continuous variables relate.
sns.scatterplot(data = df_taxis, x = "distance",
y = "fare", alpha = .5)
<AxesSubplot:xlabel='distance', ylabel='fare'>
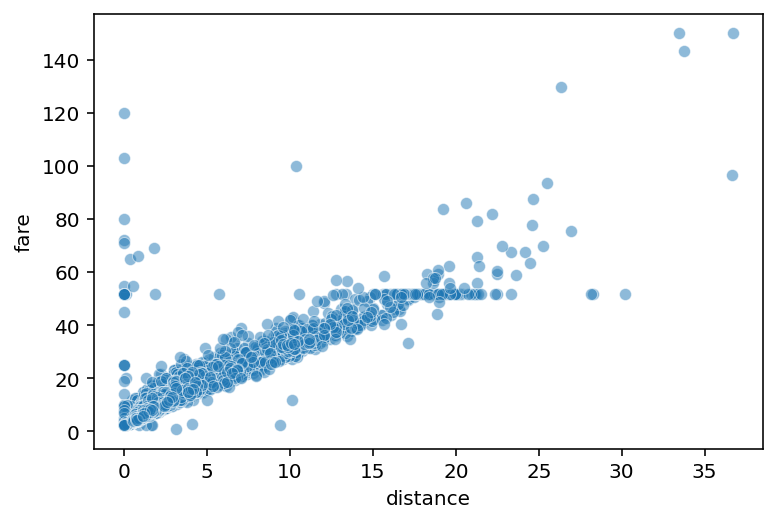
Boxplots#
Boxplots are useful for visualizing one continuous variable as it relates to a categorical variable.
sns.boxplot(data = df_taxis,
x = "payment",
y = "fare")
<AxesSubplot:xlabel='payment', ylabel='fare'>
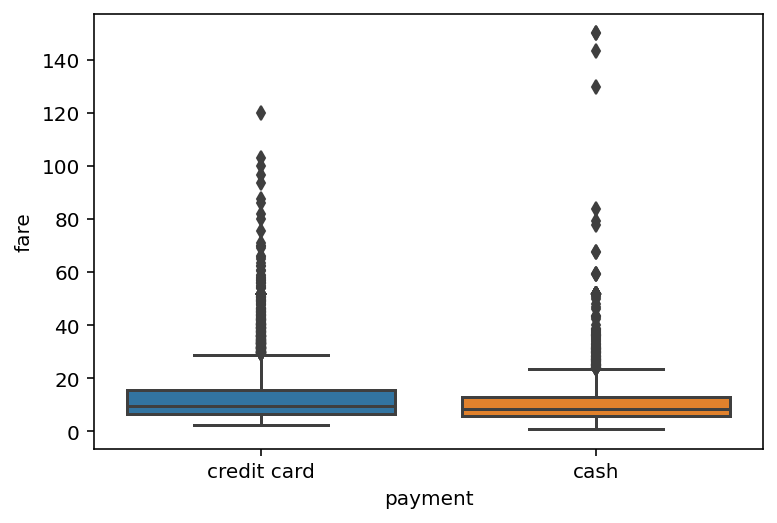
Barplots#
Barplots are also useful for visualizing one continuous variable as it relates to a categorical variable.
Typically less informative than a boxplot or violinplot.
sns.barplot(data = df_taxis,
x = "payment",
y = "fare")
<AxesSubplot:xlabel='payment', ylabel='fare'>
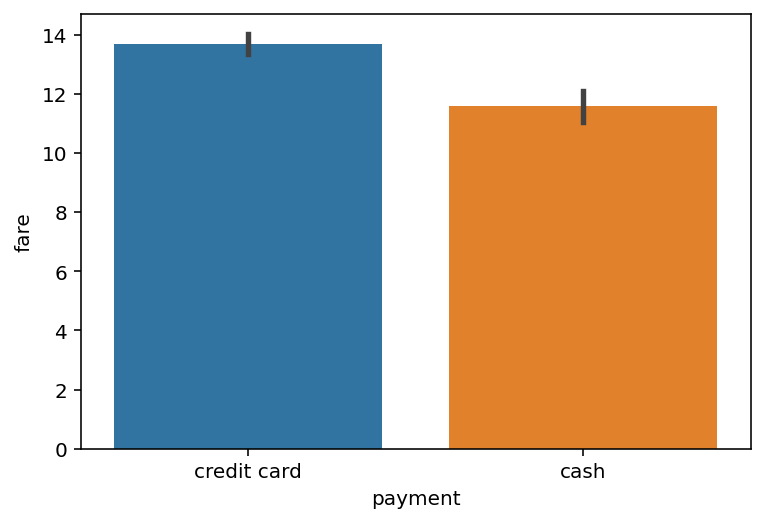
Statistics with scipy
#
scipy
is a Python package with many uses, including statistics.
import scipy.stats as ss
Calculating correlations#
scipy.stats
can be used to calculate a correlation coefficient.
## Gives correlation between distance and fare
ss.pearsonr(df_taxis['distance'],
df_taxis['fare'])
(0.9201077027895823, 0.0)
Comparing to visualization#
Consistent with correlation coefficient: strong, positive relationship between fare
and distance
.
sns.scatterplot(data = df_taxis, x = "distance",
y = "fare", alpha = .5)
<AxesSubplot:xlabel='distance', ylabel='fare'>
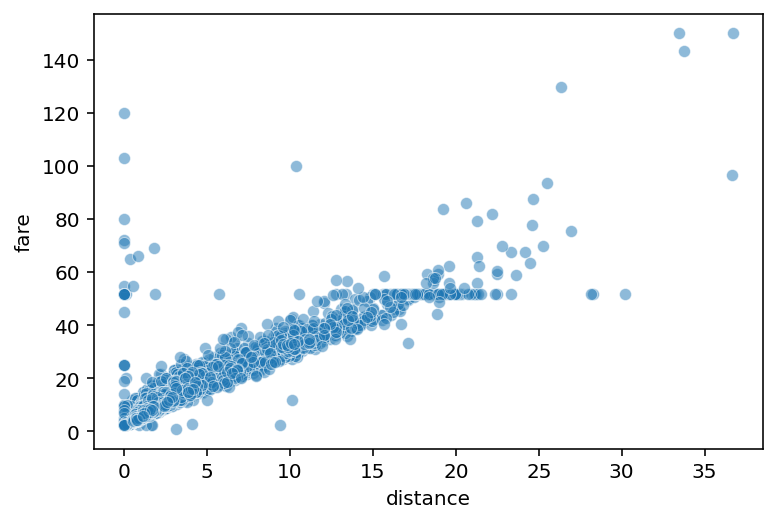
Running a t-test#
A t-test compares two samples: how likely is it that these two samples came from the same “population”?
sns.barplot(data = df_taxis,
x = "payment",
y = "fare")
<AxesSubplot:xlabel='payment', ylabel='fare'>
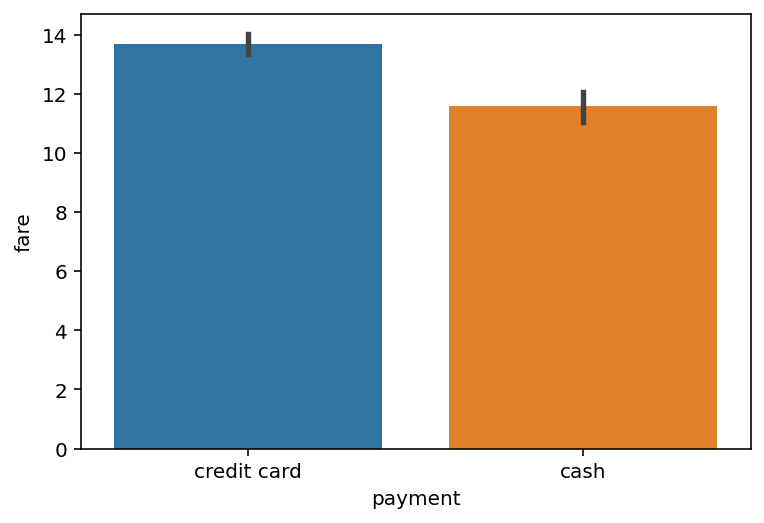
t-test in scipy
#
## First, filter our data to get cash vs. credit
cash = df_taxis[df_taxis['payment'] == 'cash']
credit = df_taxis[df_taxis['payment'] == 'credit card']
## Now, run t-test comparing fare for cash vs. credit
## Very significant p-value
ss.ttest_ind(credit['fare'], cash['fare'])
Ttest_indResult(statistic=6.584862944621184, pvalue=4.91557250875317e-11)
NLP with nltk
#
nltk
, or Natural Language Toolkit, is an incredibly useful Python package for computational linguistics and NLP.
Must be installed.
import nltk
Word tokenizing#
So far, we’ve been trying to tokenize text using
split
.Sometimes this doesn’t work easily––we have to write complicated code.
But
nltk
has written useful functions for tokenizing text for us.
nltk.word_tokenize("This is a bunch of words")
['This', 'is', 'a', 'bunch', 'of', 'words']
nltk.word_tokenize("Even with commas, it does well!")
['Even', 'with', 'commas', ',', 'it', 'does', 'well', '!']
Sentence tokenizing#
nltk
can also be used to tokenize at the level of sentences.
nltk.sent_tokenize("This is one sentence. This is another sentence. Here's another!")
['This is one sentence.', 'This is another sentence.', "Here's another!"]
Part-of-speech taggging#
Part-of-speech tagging is an operation that involves identifying whether each word is a noun, verb, etc.
Very hard to write code to do this ourselves.
nltk
does it for us!
pos_tag
with nltk
#
## First, tokenize a sentence into words
words = nltk.word_tokenize("I walked into the room.")
## Then, run pos tagger
nltk.pos_tag(words)
[('I', 'PRP'),
('walked', 'VBD'),
('into', 'IN'),
('the', 'DT'),
('room', 'NN'),
('.', '.')]
Handling words with multiple POS#
Some words can be either nouns or verbs––nltk
guesses POS based on context.
## "walk" as noun
nltk.pos_tag(nltk.word_tokenize("He went for a walk."))
[('He', 'PRP'),
('went', 'VBD'),
('for', 'IN'),
('a', 'DT'),
('walk', 'NN'),
('.', '.')]
## "walk" as verb
nltk.pos_tag(nltk.word_tokenize("He likes to walk."))
[('He', 'PRP'), ('likes', 'VBZ'), ('to', 'TO'), ('walk', 'VB'), ('.', '.')]
Sentiment analysis#
As we’ve seen, sentiment analysis involves estimating the valence of text.
Our approach has been simple: count the positive and negative words.
But sometimes a word means different things based on context (“The movie was not great”).
nltk
tries to account for this in its sentiment analysis tool.
SentimentIntensityAnalyzer
#
from nltk.sentiment.vader import SentimentIntensityAnalyzer
analyzer = SentimentIntensityAnalyzer() ## This is a *class*
analyzer.polarity_scores("That restaurant was great!")
{'neg': 0.0, 'neu': 0.406, 'pos': 0.594, 'compound': 0.6588}
Accounting for negation and punctuation#
## "not delicious" ≠ "delicious"
analyzer.polarity_scores("That restaurant was not great!")
{'neg': 0.473, 'neu': 0.527, 'pos': 0.0, 'compound': -0.5553}
## Punctuation can "intensify" sentiment
analyzer.polarity_scores("That restaurant was not great!!!")
{'neg': 0.51, 'neu': 0.49, 'pos': 0.0, 'compound': -0.6334}
There’s always a “but”#
nltk
weights the text after the “but” more than the text before.
## Punctuation can "intensify" sentiment
analyzer.polarity_scores("I liked the food, but the ambience was horrible.")
{'neg': 0.375, 'neu': 0.474, 'pos': 0.15, 'compound': -0.5927}
Conclusion#
This class is intended to set the foundation.
Now you are better set up to learn new tools in Python or other programming languages.
Many more useful tools in Python.
Thanks for a great quarter!